Picture this: It’s Wall Street in the 1980s, where ambitious traders are making split-second decisions, yelling their orders across the chaotic trading floor. Now fast-forward to 2023 – trading floors have turned eerily quiet as cutting-edge technology has transformed the industry with mind-blowing precision and speed. Enter automated trading algorithms – the driving force behind this silent revolution. In this blog post, we’ll dive deep into how these digital masterminds have unlocked the true potential of trading and shaped a new era of financial innovation that will blow your socks off! But first, buckle up as we take you on a thrilling ride comparing high-octane Formula One racing with the exhilarating world of algorithmic trading.
Trading algorithms, also known as algorithmic trading or algo-trading, use mathematical models and predefined rules to analyse market data and automatically place trade orders. These programmes can identify trends, patterns, and other indicators that traders could potentially miss with manual analysis. By removing human emotions from the process and executing trades at high speeds, algo-trading can help increase efficiency and profitability for investors.
Table of Contents
Trading Algorithm Functionality
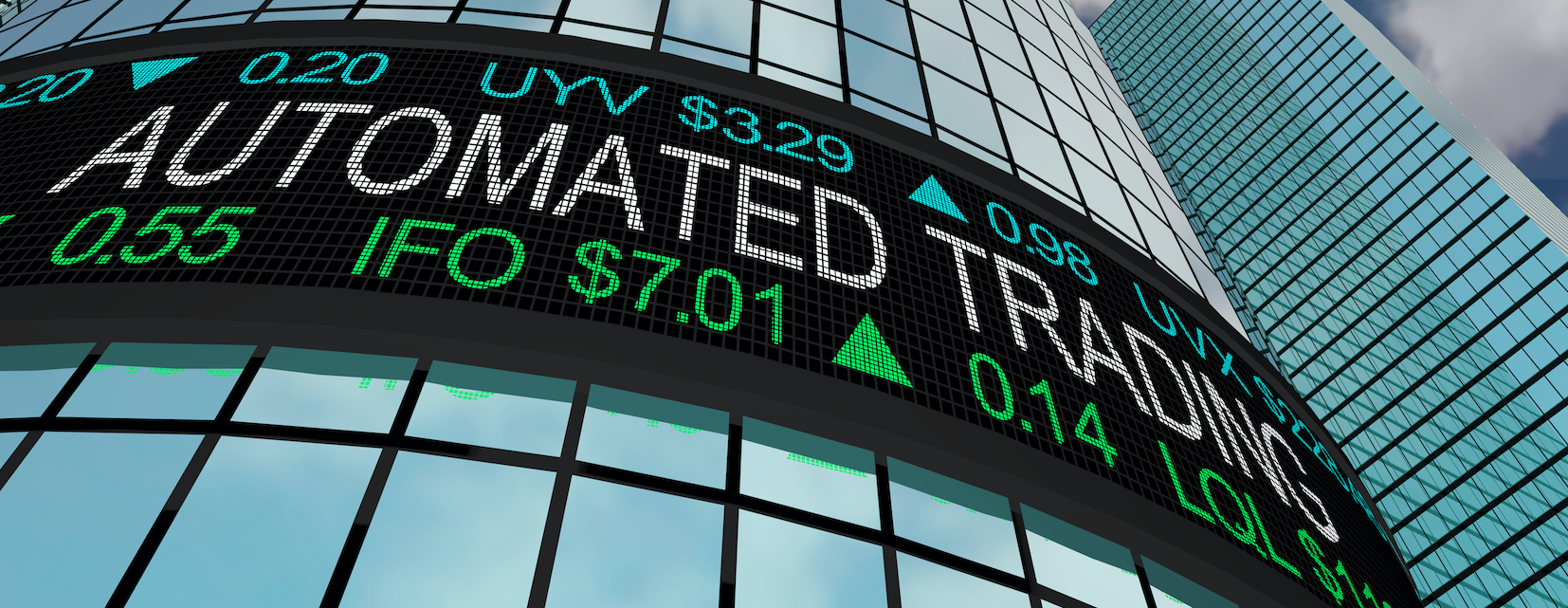
Automated trading using algorithms provides numerous advantages for traders, who are looking to eliminate the emotional aspect of trading and optimise their decision-making process. By relying on computer programmes to make buying and selling decisions based on preset rules, investors can benefit from speed, accuracy, and efficiency in executing trades. Algorithms come in various forms and features, but they all share some basic functionality that makes them suitable for day-to-day trading.
The primary concept underlying algorithmic trading is automation. An algorithm is a set of predefined instructions that a computer programme uses to execute specific functions without human intervention. Algo-trading allows investors to automate their investment strategies by setting rules for when to enter or exit a position based on technical or fundamental indicators. For instance, an algorithm can be programmed to buy a stock only when its 50-day moving average crosses over the 200-day moving average.
One example of how algorithms can improve trading performance is through the use of limit orders. A limit order is an instruction given to a broker to buy or sell a security at or below a specific price. Limit orders can be used to minimise slippage, which occurs when the market moves against an investor’s position while an order is being executed. By using algorithms to manage limit orders automatically, traders can ensure better execution prices without having to monitor prices constantly.
Another essential feature of algorithmic trading is backtesting. Backtesting involves testing historical data against parameters of an algorithm to analyse how it would have performed under different market conditions in the past. Traders can use backtesting effectively by analysing the performance metrics of different algorithmic models and optimise their strategies accordingly.
Algo-traders also rely on algorithms’ scalability as one major advantage because many strategies require considerable capital efficiencies for profit generation. Algorithms provide a high degree of scalability because they can handle multiple positions and trade volumes simultaneously without compromising the execution speed or accuracy.
Moreover, to further enhance the scalability of their strategies, algo-traders can leverage cutting-edge trading platforms like Immediate Connect. This innovative platform seamlessly integrates with algorithms, offering robust scalability features that allow traders to efficiently manage multiple positions and trade volumes concurrently. Immediate Connect ensures that execution speed and accuracy remain uncompromised, enabling traders to maximise their profit potential while capitalising on considerable capital efficiencies.
Automated Process and Decision Making
Algorithms’ primary function in trading is to automate the decision-making process, which can help traders avoid emotional bias and impulsive behaviour that might negatively affect trade outcomes. Automated trading enables traders to test market hypotheses in real-time by using rule-based trading systems where pre-set conditions trigger market entry or exit decisions.
Automated trading operates around a few fundamental principles. First, automated systems need access to reliable data sources that provide accurate and timely information about financial instruments. This data can be obtained from various sources, such as stock exchanges, financial news providers, or specialised data vendors that collate market data.
Next, automated trading requires well-defined criteria to initiate trades based on specific market indicators. For instance, technical analysts use charts and indicators like moving averages and trendlines to identify current price trends and points of entry or exit from a position.
The third critical aspect of automated trading is order routing. Order routing refers to the process of sending orders generated by an algorithm to the appropriate exchange or market maker for execution. Order routes are created using pre-defined routing logic that considers various factors impacting order execution, such as liquidity, spreads, fees, and market impact.
Some critics argue that automated trading systems ignore important contextual information about the markets’ current state and may fail to account for unforeseen events. They also suggest that “black-box” algorithms are opaque and may not be able to reflect changes in investor sentiment or broader economic conditions properly.
However, proponents of algorithmic trading contend that sophisticated algorithms can adapt dynamically based on real-time market data streams and adjust their strategies accordingly. They argue that algorithmic models can sift through vast amounts of incoming data and extract relevant features using machine learning techniques.
Implementing AI in Trading
Automated trading systems have effectively streamlined the trading process by increasing efficiency and reducing human error. However, algorithms on their own can only do so much. Enter AI, which can take trading integration to the next level.
One example of implementing AI in trading is through natural language processing (NLP), which involves analysing news articles and social media sentiment for indicators that may impact stock prices. Another implementation of AI is in pattern recognition, which can identify patterns in data that may be too complex for humans to detect. This information can provide traders with a competitive advantage and improve returns.
Analogously speaking, implementing AI in trading is like having a highly experienced trader who has years of experience, always alert and never misses a beat – but without requiring a paycheck or breaks. The machine learning algorithms are constantly working tirelessly behind the scenes to analyse market trends, forecast future changes and identify opportunities for investments.
AI’s capability to learn from past experiences and adapt to new market conditions means that trading algorithms will continue to evolve and improve over time, giving traders an edge over those who rely solely on instinct and human decision-making.
Anecdotal evidence shows that AI integrated with automated trading has yielded significant benefits for firms that embrace it. One major institutional investor implemented machine learning algorithms with its existing trade execution algorithms, resulting in a 27% increase in trades executed within the best bid/ask spread. Additionally, they observed a decrease in “market impact costs,” referring to the disruption in stock prices caused by large transactions in thinly traded markets.
There are also studies that demonstrate how deploying AI into trading systems enhances investment performance. A team of researchers at MIT analysed hedge fund data from 1994 to 2014 and found that funds utilising machine learning outperformed their counterparts. Furthermore, algorithmic trading systems using AI were able to achieve annualised returns of 34%, which is much higher than the S&P 500’s long-term average.
However, like any tool, AI in trading has its drawbacks. One potential concern is that AI’s decision-making process is based solely on what it was trained to do. Therefore, if the machine learning algorithms are built around a bias or flaw, it may lead to incorrect predictions and results. Additionally, there is always a risk that relying too heavily on technology can cause traders to overlook market indicators that would otherwise be picked up by human intuition.
Pros of Using Trading Algorithms
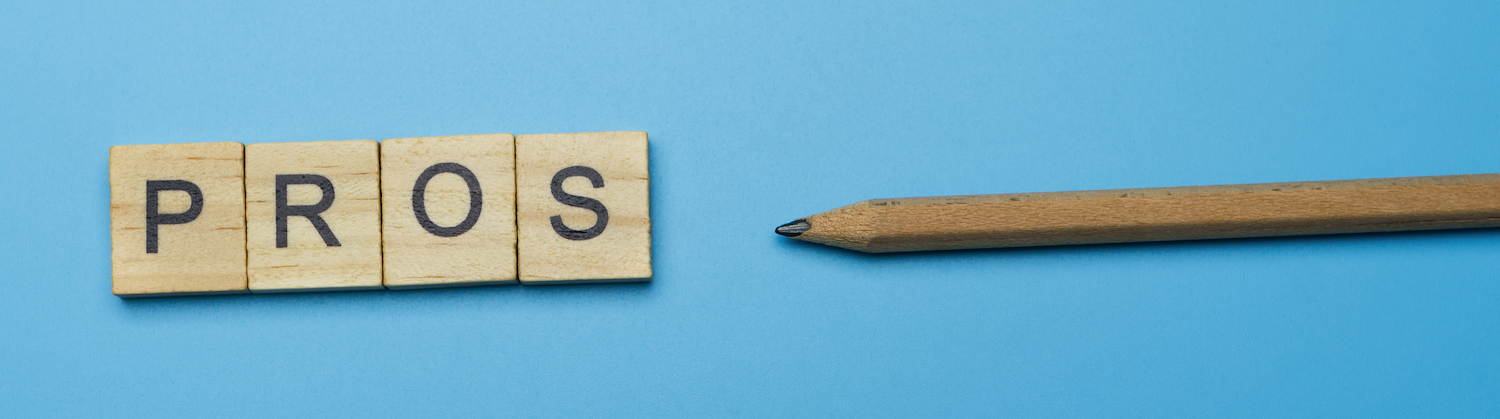
Despite some of the concerns surrounding algorithmic trading, there are many benefits to automating trades in financial markets.
First and foremost, trading algorithms remove emotions from the equation. Humans can often make irrational choices based on fear and greed when it comes to investing. In contrast, algorithms make logical decisions based on historic and real-time data analysis alone.
Another advantage of using trading algorithms is their ability to execute trades at a high speed. This means that investors can take advantage of price discrepancies between different markets or exchanges before human traders even realise they exist.
For instance, HFT firms have been able to narrow bid-ask spreads considerably from fractions of cents down to thousandths or even millionths of a penny through sophisticated algorithmic strategies while also reducing costs due to fewer human personnel required for maintaining these systems and increased efficiency.
Studies have shown that automated trading systems perform better than manual trading, particularly in terms of minimising transaction costs and achieving best execution. In addition, algorithmic trading allows individual investors access to trade like an institutional trader – for example, having access to dark pools and other liquidity sources inaccessible to retail investors when using direct market orders.
On the other hand, one disadvantage of algorithmic trading could be related to regulatory requirements. Developments in the area of algorithmic trading have led to regulatory updates intended to ensure that trading is conducted fairly and efficiently. A challenge that investors using algorithms may face is complying with these regulations, as breaking them may result in significant legal fines and reputational damage to one’s firm.
- Automating trades in financial markets through trading algorithms has various benefits such as removing emotions and making logical decisions based on historic and real-time data analysis, executing trades at high speed to take advantage of price discrepancies, performing better than manual trading in minimising transaction costs and achieving best execution, and allowing individual investors access to trade like an institutional trader. However, regulatory requirements may pose a challenge for investors using algorithms to comply with these regulations to avoid significant legal fines and reputational damage to their firms.
Cons of Using Trading Algorithms
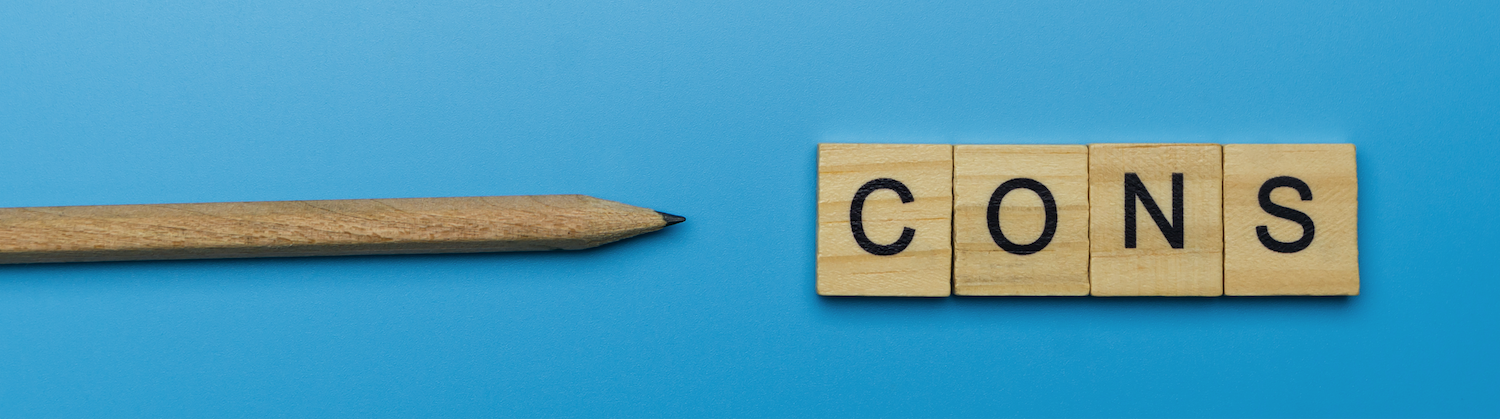
While automated trading comes with many advantages, there are also several drawbacks that cannot be ignored. It’s important to consider these cons before investing in algorithmic trading. Here are some of the issues that traders and investors might face when using this type of strategy.
One of the biggest disadvantages of using trading algorithms is the risk of technology failure. While algorithms are designed to trade effectively, they can only perform as well as the technology allows them to. If there is a glitch in the system or a connexion error, trades may not be executed on time or at all. This can lead to significant losses for traders who rely solely on algorithms.
In addition to technology failures, another con of algorithmic trading is market impact. When large numbers of orders are placed simultaneously by traders using similar algorithms, it can cause market imbalances that can seriously affect asset prices. This is particularly true for high-frequency trading (HFT), where trades take place at exceptionally high speeds.
Some critics argue that algorithmic trading can also lead to an increase in volatility in the markets. According to this view, computer programmes tend to amplify small price movements by triggering large numbers of buy or sell orders almost instantaneously. Because algorithms don’t take into account fundamental economic factors and news events, their decisions can sometimes be divorced from reality. However, others argue that this type of trading actually helps smooth out volatility by providing more liquidity and enabling trades to be executed more efficiently.
Investors should also be aware that while algorithms are based on historical data and statistical models, they cannot predict future events with complete accuracy. Even if an algorithm has been backtested and shown impressive results, it doesn’t mean it will work perfectly in current market conditions. There is always a risk that unforeseen changes in market dynamics could negatively impact the performance of an algorithm.
To put it simply, algorithmic trading is like driving a car on autopilot. While the technology behind self-driving cars has come a long way, they are not yet foolproof. As with algorithms, there’s always the risk that something could go wrong while you’re cruising down the highway. That doesn’t mean you shouldn’t use self-driving technology, but it does mean you need to remain vigilant and aware of potential risks.
Algorithm Types and Strategies
Despite the potential drawbacks of using algorithmic trading, this approach can still be an effective way to manage investments. There are a variety of types of algorithms and strategies available to traders, each with its own strengths and weaknesses. Here are some examples:
One popular type of strategy is trend-following, where algorithms analyse price trends over time to determine whether an asset is expected to appreciate or depreciate in value. This type of strategy can be particularly effective in markets that exhibit clear directional trends.
Another type of algorithmic strategy is arbitrage, which involves identifying price discrepancies for identical or similar assets across different markets. Traders can then profit from these discrepancies by buying low in one market and selling high in another.
Multi-factor models are another popular approach that relies on a comprehensive analysis of various factors that affect asset prices. These models take into account economic data, company financials, news events, and other variables in order to generate trades that are based on more than just past price movements.
Finally, many traders choose to develop their own customised algorithms based on unique market insights or specific trading goals. By designing algorithms tailored specifically for their needs, traders can increase their chances of success in the market.
Overall, there is no one “right” way to implement trading algorithms. The choice will depend on a trader’s individual goals, market conditions, and risk tolerance. It’s important to do your research and understand the pros and cons of each algorithmic approach before integrating them into your trading strategy.
Arbitrage, Trend-following, and More
As mentioned in the previous section, trading algorithms offer a range of functionalities to traders. They provide an effective way to automate and streamline the trading process for better results. One of the key advantages of using trading algorithms is the ability to implement different types of trading strategies. These strategies enable traders to make informed decisions based on predefined rules and criteria, which can be adjusted according to market conditions. In this section, we will delve into some popular algorithm types and strategies that traders often rely on.
Arbitrage
One classic trading strategy is arbitrage. It is based on exploiting price differences between two or more markets. The idea is to buy an asset at a lower price in one market and sell it at a higher price in another market simultaneously. With algorithmic trading, arbitrage opportunities can be quickly identified and exploited since the programme can perform trades instantly across multiple markets.
For instance, let’s say that Nike stock is traded at a lower price on NASDAQ compared to its price on NYSE. An arbitrageur would buy Nike stock on NASDAQ while simultaneously selling it at a higher price on NYSE thus making a profit from the difference in prices.
Trend-following
Another common strategy used with algorithmic trading is trend-following. This strategy is based on identifying trends in asset prices through chart analysis and predicting whether they are likely to continue or reverse. By following trends, traders seek to capitalise on significant price movements.
Trend-followers usually employ technical analysis tools such as moving averages and relative strength indexes (RSI) to identify trends and momentum of an asset. For example, if there’s been a sustained uptrend in Apple stock over several weeks, or months then traders might use an algorithmic approach like trend-following that uses pre-defined triggers based on charts to execute trades based on these observations.
Mean Reversion
Contrary to trend-following, mean reversion aims to identify overbought and oversold assets. This strategy is based on the principle that prices tend to return to their mean value after reaching extreme levels. Under this approach, traders buy when assets are undervalued and sell when they are overvalued expecting the prices to move back towards the average price range.
For example, let’s consider an asset that has historically traded in a certain price range but spikes up or down unexpectedly. Mean reversion traders would wait until the asset returns to pre-event price levels before executing trades mathematically.
Strategy Implementation
It is important to note that while algorithms can be programmed with a specific trading strategy, these programmes should not be seen as a silver bullet solution for investing. The accuracy and success of decisions made by an algorithm rely on the quality of data inputs, market volatility, and other factors outside the control of traders.
Additionally, some strategies may work better in certain markets than others. For instance, a trend-following strategy might be appropriate for trending markets but may not perform as well in sideways or choppy markets.
Backtesting
To mitigate potential risks of employing flawed strategies or poor-quality input data, traders often rely on backtesting these strategies against historical data with the help of algorithmic trading. This allows them to simulate the performance of any particular trading strategy over a period before putting it into action in live trades.
Backtesting provides insights into how different strategies have performed in varying market conditions which gives confidence and risk protection when using automated algorithms.
In summary, there are several types of algorithmic trading strategies that can be implemented through automated algorithms such as arbitrage, trend-following, mean reversion and more. While these strategies have some benefits such as fast execution time, cost efficiency, and scalability, traders should be cautious when employing them and ensure that strategies have been adequately backtested and are compatible with current market conditions.